December 10, 2024
Rethink AI governance by treating models like programs, not just data
See Liquibase in Action
Accelerate database changes, reduce failures, and enforce governance across your pipelines.

Your pipeline’s embedded AI/ML (artificial intelligence/machine learning) capabilities, internal AI tools, and customer-facing AI-driven apps are part of a new era of innovation, powering insights, predictions, and products that reshape entire industries.
But is the data fueling them good enough – safe, accurate, secure, complete enough – to maintain your reputation of reliability while pushing new frontiers of technology?
That data going into AI pipelines and products is the make-or-break point. Yet governing that AI data is often only approached as a safeguard against errors, risks, and compliance – critical elements, of course, but not getting at the full value of AI investments up and down the pipeline.
Instead, AI governance must be approached as a:
- Foundation or trust (in your data, AI, and company overall)
- Catalyst for innovation and optimization
And to make that switch from a narrow focus on safety to a more comprehensive embrace of reliability and value, teams managing AI pipelines need to treat AI/ML models not as static datasets but as dynamic programs. Thinking of them in the same sense as software application development pipelines allows the integration of the same DevOps-aligned tactics of versioning, testing, and monitoring.
AI governance isn’t just about preventing bad outcomes — it’s about enabling good ones.
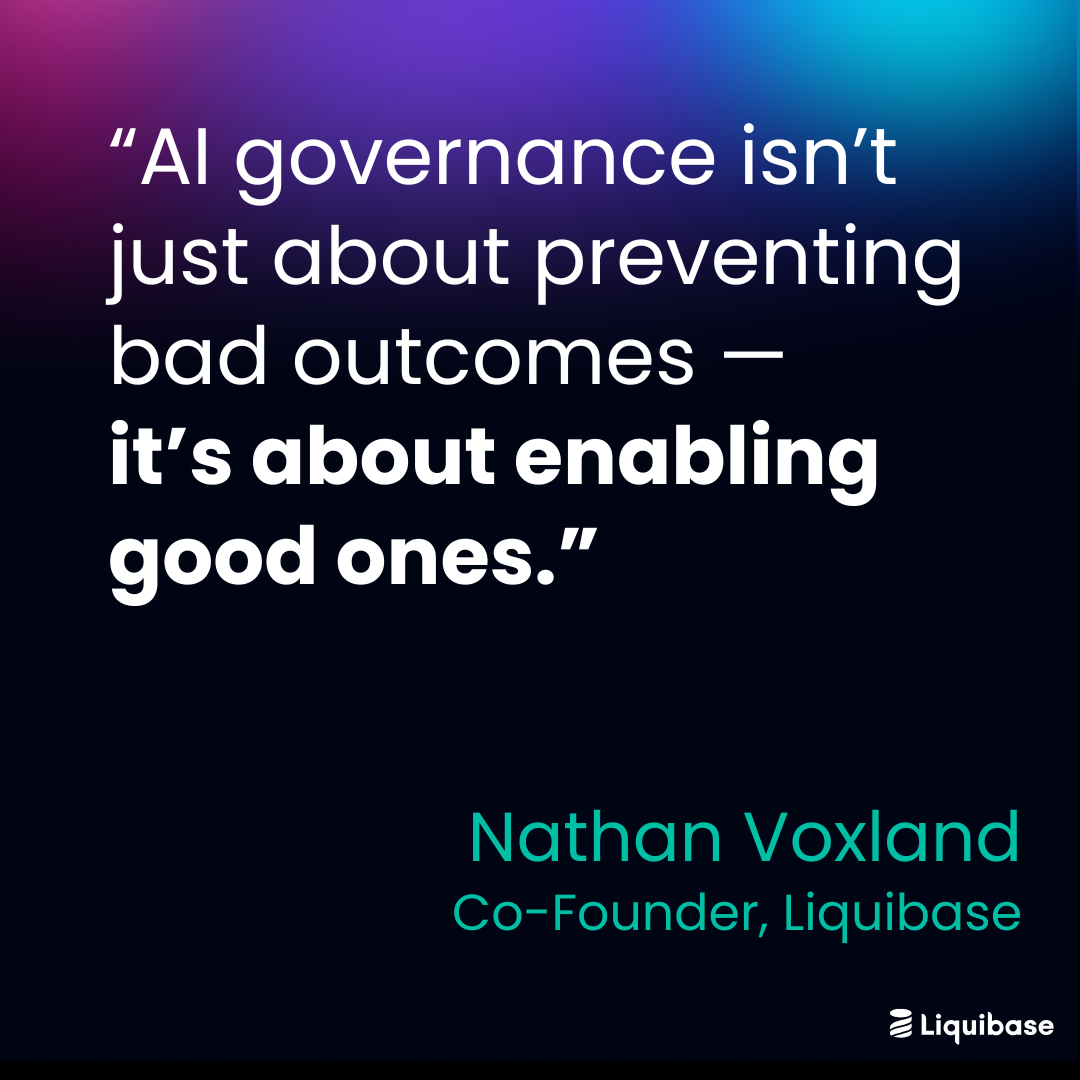
Every AI model is a product of its training process, requiring the same rigor as any other software. Without robust governance, small changes in upstream data can cascade into massive disruptions downstream, eroding the trust of end users and undercutting the value of AI investments.
By rethinking governance with a programmatic mindset, organizations can unlock the full potential of their AI systems. Through structured lifecycle management, proactive monitoring, and scalable data practices, governance shifts from a hurdle to a competitive advantage. This approach empowers teams to innovate faster, adapt to change, and maintain the trust that is essential for delivering impactful AI products.
Shifting perspective: AI models as programs, not (just) data sets
Here’s the crux: AI models are not static objects to be trained once and left alone.
Instead, they are dynamic living programs built on data that evolves over time. Just as software requires version control, testing, and monitoring to ensure reliability and scalability, AI models need governance processes that adapt to the iterative nature of their development.
Whenever there are even small updates, transformations, or restructurings to upstream data, there can be a cascade of impacts on AI outputs. How are those changes being tracked and what happens when there’s a disruption? Will it go unnoticed – until it's disastrous – or uncatchable? Without proper governance, these disruptions may go unnoticed until they surface in production, where they’re far more costly and damaging to address.
The key is to treat AI models like any other critical software component. Lifecycle management should include versioning for datasets and models to ensure that every iteration is documented and reproducible. Regression testing becomes vital, allowing teams to identify how changes in data or training parameters impact performance. By embedding these principles into AI governance, organizations can proactively detect and mitigate risks, ensuring that AI outputs remain consistent and trustworthy over time.
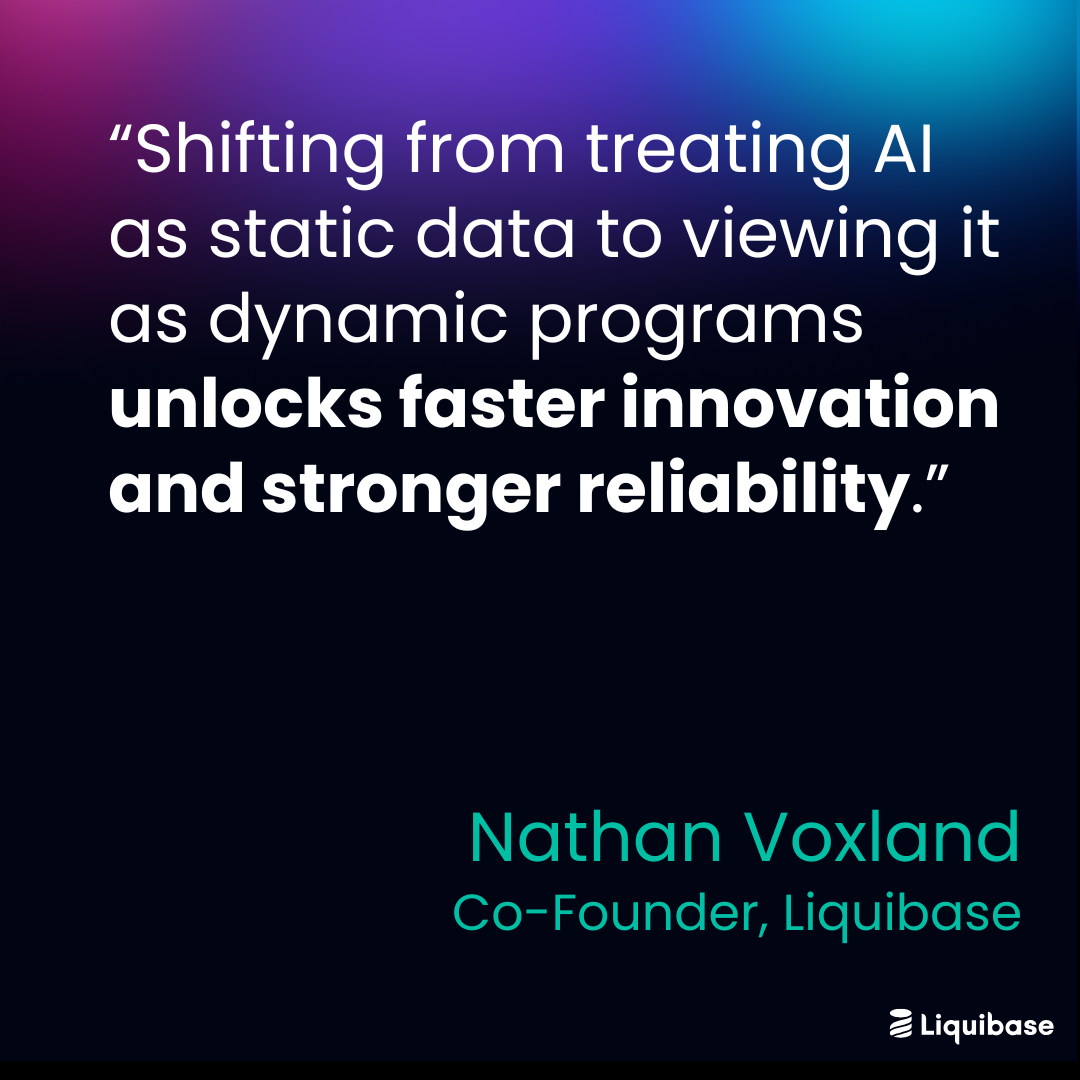
This shift in perspective — from treating AI as “just data” to viewing it as a dynamic program — empowers teams to move faster while maintaining control. Governance doesn’t have to be a bottleneck, but instead a strategic enabler for delivering impact and upholding reliable standards for future AI-driven innovations.
Build trust in AI outputs through continuous feedback
This isn’t to say AI governance is a one-and-done task, of course. There’s more to making the switch than just a switch in perspective. But to go any further requires visibility and understanding.
Models don’t just operate on static data — they continuously evolve with new training datasets, parameters, and use cases. This iterative nature demands ongoing monitoring to ensure outputs are consistent and improving. Monitoring and feedback loops are essential because you need to know if the AI outputs are improving or degrading over time and act accordingly.
By embedding robust monitoring mechanisms, organizations can proactively identify and address inconsistencies before they impact the users, reputation, or bottom line. This builds trust not only in the models themselves but also in the broader AI initiatives driving innovation. Reliable governance processes give teams the confidence to iterate quickly while maintaining quality and reliability.
Automating AI governance for scalability
Then that confidence has to extend to every pipeline and every player up and down the data pipeline.

Scaling AI governance for dynamic, enterprise-level pipelines is impossible without automation. AI models are different from regular software in that they’re created by training, not coding. Governance needs to handle versioning, testing, and monitoring of these training models, just like components in traditional software.
Automated workflows enable the reproducibility, scalability, and traceability across the AI pipeline teams need to put version control, monitoring, and feedback loops into action. By integrating governance into the development process, organizations can enforce policies, track provenance, and ensure datasets meet compliance and quality standards.
Automation transforms governance from a manual, error-prone process into a streamlined foundation that empowers teams to innovate confidently at scale. With automation as a cornerstone, AI governance shifts from a limiting requirement to an enabler of trust and agility, driving innovation without sacrificing reliability or compliance.